Machine Learning Search Bot reduces risk for brands and saves costs
Working with stakeholders, I designed a Search Bot that employs Machine Learning to monitor ICUC clients’ social media feeds and protect their brands from unforeseen events.
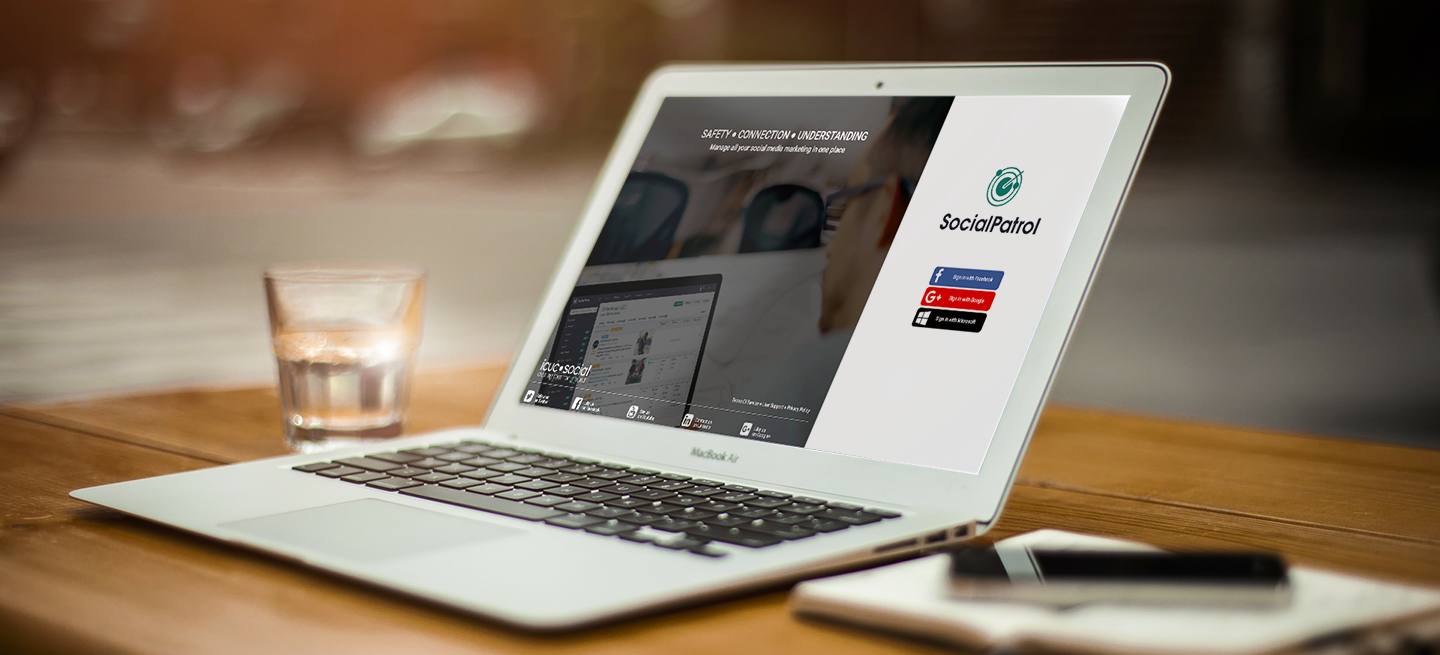
Project team and my responsibilities
I was part of a five-member project team: Machine Learning Software Architect, Front-end Developer, Machine Learning Analyst, Product Owner, and UX Designer (me). We were all part of a remote 25-member Agile product team.
I was responsible for designing the search bot feature. My process included research, refining the problem, UI design, interaction design, prototyping and testing.
Challenge
SocialPatrol is an enterprise web app developed by ICUC to manage their clients’ social media channels. SocialPatrol clients needed a way to monitor their social media feeds for extraordinary once-in-a-blue-moon events. ICUC needed to reduce the risk of missing positive or negative exceptional posts and help content specialists respond faster and more accurately to such posts.
Value for users, clients and ICUC
For users responsible for managing clients' social media content, this feature would act as a safety net, ensuring that posts about unprecedented events are not missed and improving response time.
For clients, this feature would save brands from the fallout of controversial and damaging posts and identify opportunities to build brand engagement with positive posts.
For ICUC, the search bot feature would reduce labour costs and ensure they meet contractual requirements.
Who would use the Search Bot, and how it would work
The search bot had two parts: creating a search and responding to content found by the search. Machine Learning Analysts and Brand Managers set up searches, and content specialists responded to content retrieved by the search.
UX process and deliverables
Discovery
The key to any successful project is understanding the problem. The team needs to understand why we needed this feature and what it needed to do.

Planning and Design
I regularly collaborated with my team throughout the project using wireframes and prototypes. I refined the design using feedback from users and the project team.

User Stories and Task Flows
I created User Stories, Scenarios and Task Flows to help the team empathize and better understand what our users needed to accomplish.
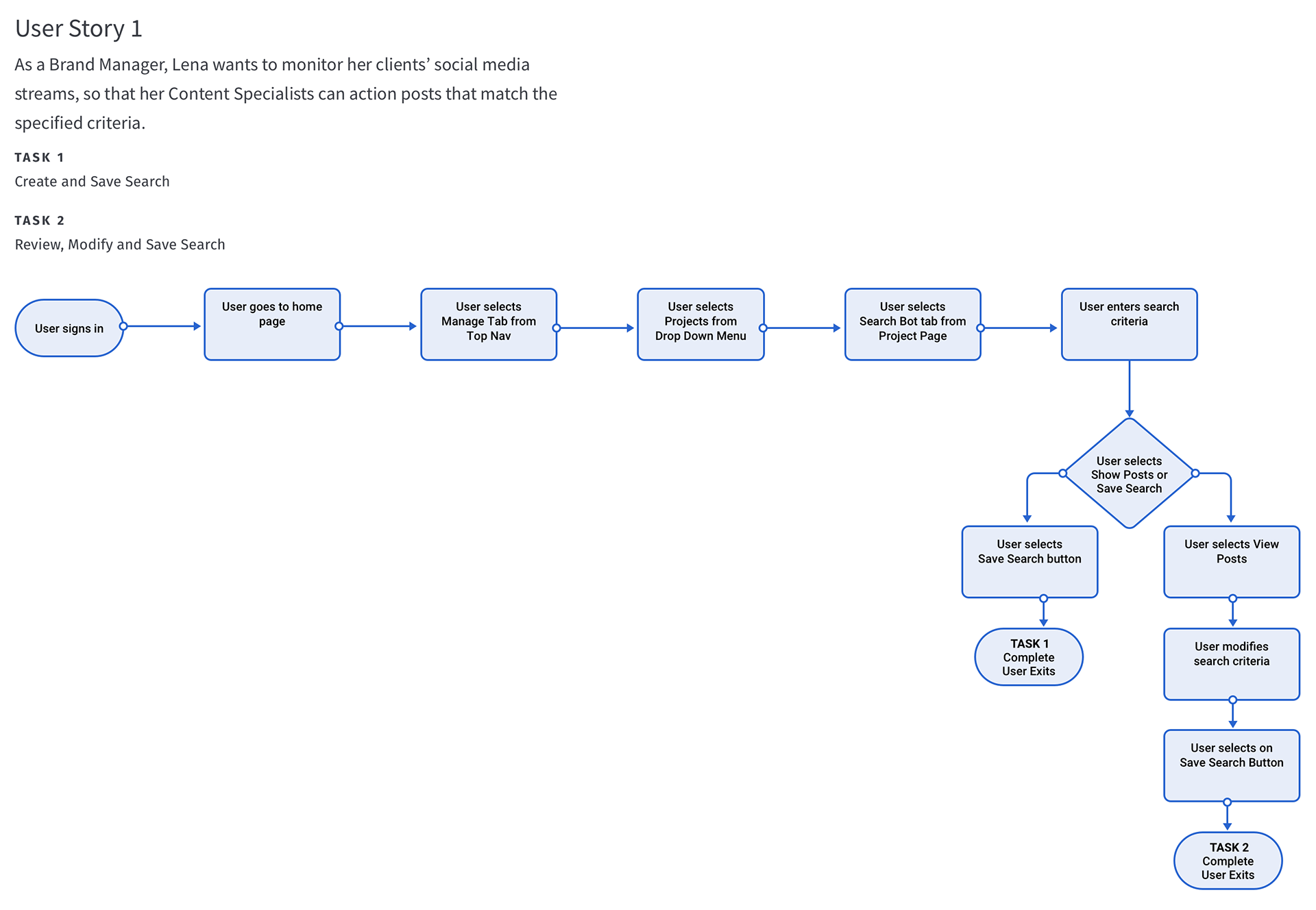
Evolving the design through wireframes
The software architect created the initial wireframe. I used that as a starting point and worked with stakeholders to clarify and identify why we were building the Search bots and what this feature needed to do. Using this information, I refined and streamlined the design. The final wireframe included enough information to efficiently complete the task and translated the terminology from developer speak to more user-friendly terms.
I designed the UI and interactions and created low and high-fidelity wireframes using Sketch. I shared the final UI design and interactions with the front-end developer using screen annotations screens and InVision’s inspect feature.
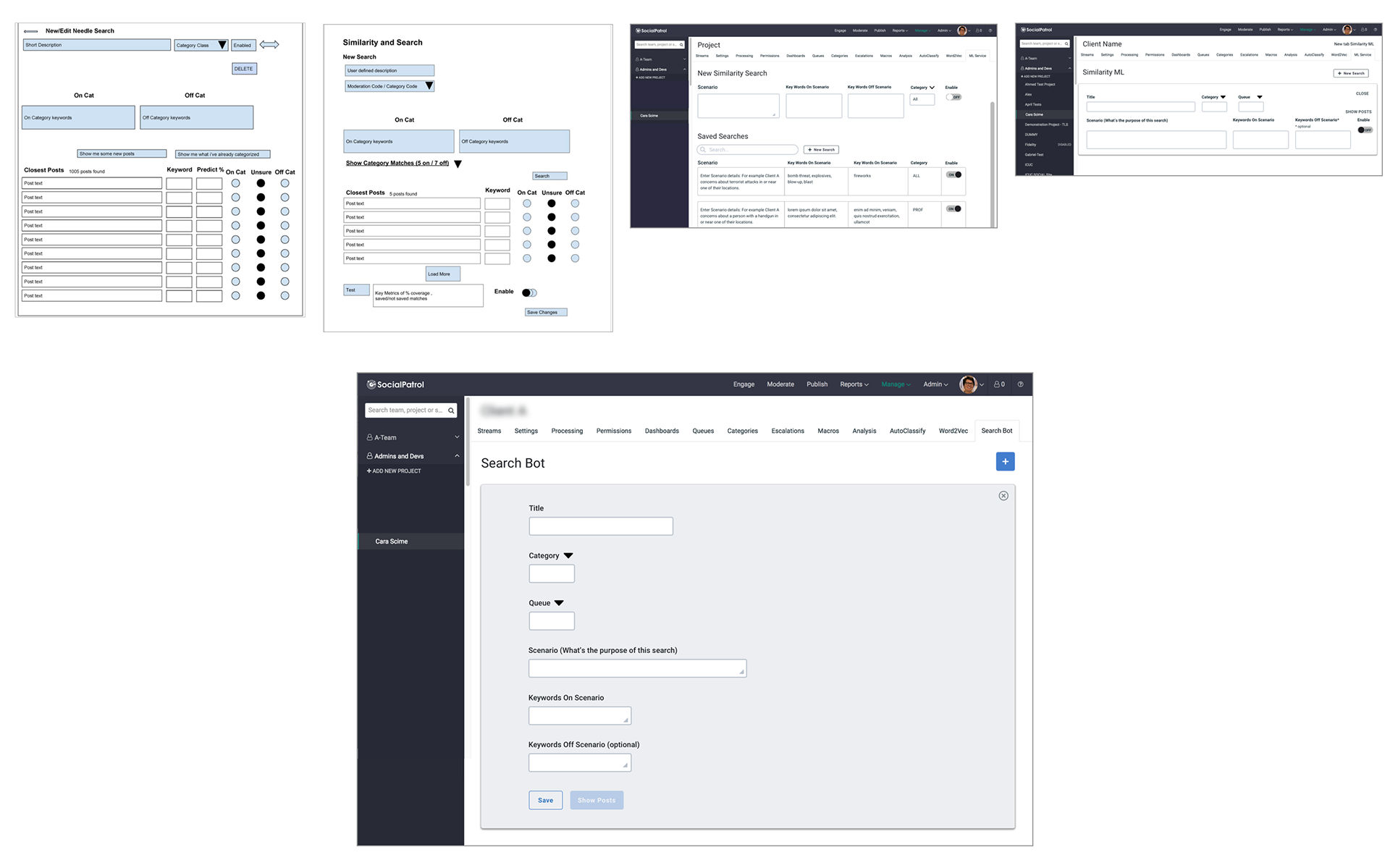
Prototyping and Testing
Prototyping and testing were key to delivering a great feature; the feedback from this stage helped us maintain focus on business and user goals. I created and tested concepts using prototypes built in InVision.
Lessons Learned and Outcome
As a designer, it can be uncomfortable to show rough ideas. Failing early was the key to our success. Showing the wrong solution guided us to build the right solution.
Early on in the project, there was confusion about how the search bot would work. How would the content specialist respond to posts highlighted by the search bot?
I created a low-fidelity prototype, and when I shared it with the team, we saw a problem. There was a disconnect between what the user and the system needed. Collaboratively, we were able to refine our solution to work for both.
At the end of our sprint, ICUC shifted its focus and put the search bot feature on hold.